KEY POINTS
- Machine learning models unlock optimal operational parameters, reduce cost of supply
- Analytics help drilling crews gain more than 60 feet per hour in vertical section of wells
- Project's potential extends beyond drilling to completion designs, reservoir performance
ConocoPhillips engineers and analytical experts are leveraging big data, advanced data analytics and machine learning to optimize the company’s Lower 48 drilling operations and more.
Drilling terms
Rate of penetration (ROP): The speed at which the drill bit can break the rock under it and thus deepen the wellbore.
Bottom hole assembly (BHA): The lower portion of the drillstring where the drilling motor is located. BHA motors can fail prematurely because of harsh drilling conditions, so being able to predict when they will fail can reduce tool-related downtime.
Seeking data-driven efficiency gains to lower cost of supply and increase production, the Gulf Coast & Rockies (GCR) Wells team kicked off a multi-phase optimization initiative in 2020 in the company’s Eagle Ford asset in South Texas, initially focusing on predicting drilling-motor failures and accelerating the rate of penetration (ROP) while drilling.
During these tests, drilling crews successfully used data analytics and machine learning to reduce premature drilling-motor failures on the bottom hole assembly (BHA) by 65 percent and increase the vertical rate of penetration by more than 60 feet per hour, a 20 percent increase.
Building on success: A multi-phase project that continues to evolve
Developed by a project team consisting of ConocoPhillips engineers and analytical experts, this data-driven project is using machine learning, artificial intelligence and data modeling to optimize operations. The initiative — a collaboration between Gulf Coast & Rockies (GCR) Wells, GCR Development, Global Wells and IT — emerged from an ideation introduced by the Eagle Ford Drilling Team during the company's 2018 Analytics & Innovation Symposium. It was originally known as the LETTER P, which stands for Leveraging Emerging Technology To Enhance Reliability -- with the P standing for predict, prevent and perform.
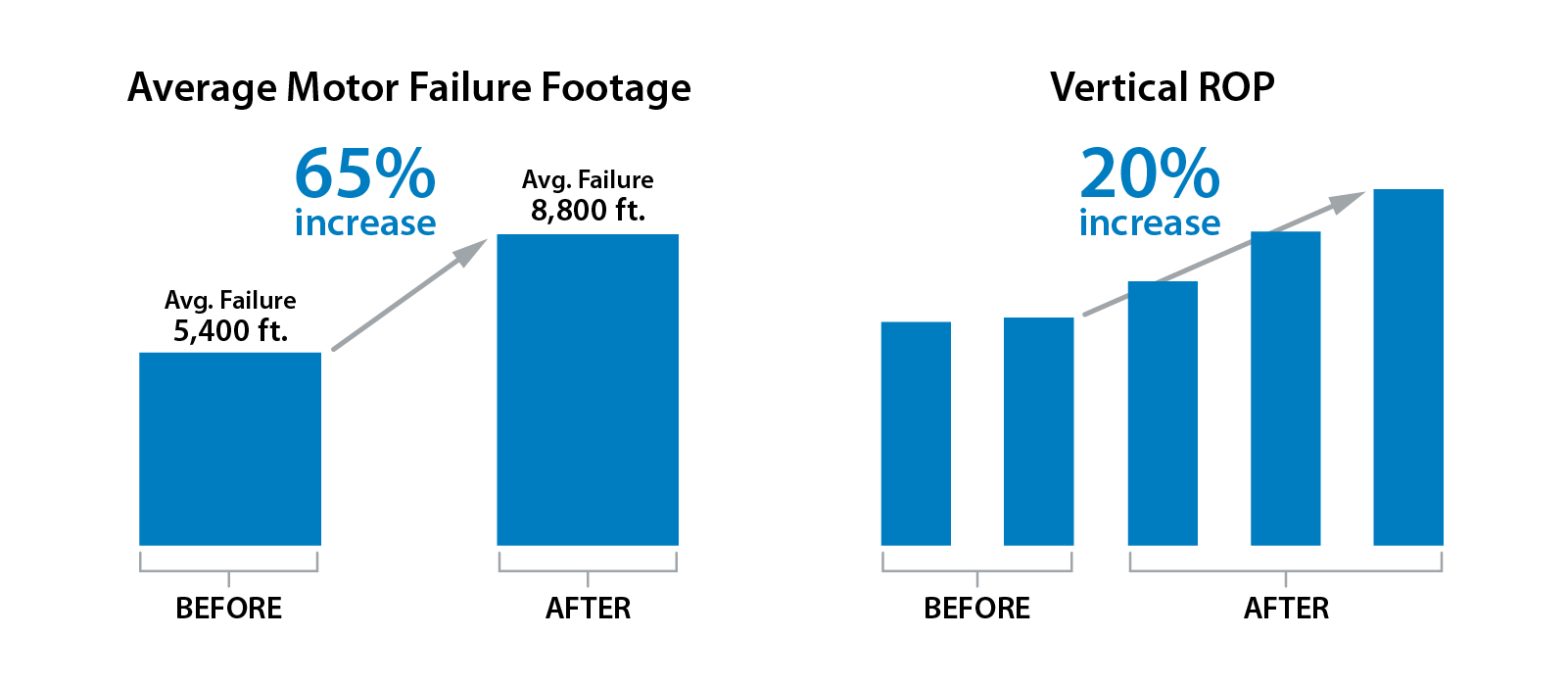
Reducing cost of supply
Since implementing this data-driven initiative, the GCR Wells team has saved more than $3 million in drilling costs in the Eagle Ford — approximately $30,000 per well, with drilling crews setting multiple speed records for drilling vertical sections since its inception.
“We recognized this as a significant cost-saving opportunity for the Eagle Ford drilling team,” said GCR Wells Completions Engineering Supervisor Justin Garner. “But we believe the prize is much bigger if we leverage this technology across all Wells disciplines.”
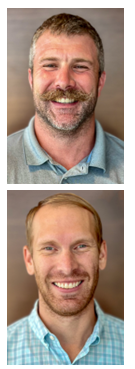
Quality data is crucial for creating an effective machine learning model
For ROP optimization, the team created a machine learning model using historical well performance data, electronic drilling recorder (EDR) data and spatial/geological data to predict the optimal operating parameters.
Electronic Drilling Recorder (EDR) data An onsite computer monitors and records measurements of all crucial drilling functions, such as weight on bit, rate of penetration and other data.
- RPM top drive
- Torque top drive
- Weight on bit
- Pump pressure
- Flow rate
- Differential pressure
- Rate of penetration (ROP)
Spatial/geological data
- Spatial/geological
- Formation tops
- Measured depth
- Easting/Northing
- Gamma ray
- Inclination
To optimize ROP, the analytics team used three years of drilling data to develop a machine learning model — using a final dataset of 116 wells and more than 138,000 observations — that balanced predictability and interpretability.
“We were able to create a highly accurate model that predicts what parameters we need to run to get the best rate of penetration,” said Eric Muller, Analytics Engineering Lead, GCR Wells. “Those parameters were then rolled out to the field. We saw an increase of 20 percent in our vertical rate of penetration almost overnight.”
The GCR Wells team hasn’t seen any negative impacts, such as an increase in BHA failures, in the vertical sections that were drilled at an increased speed, Muller said. Building on their vertical ROP success, the team is now working to increase ROP in the lateral sections of its wells.
Going forward, the GCR Wells team plans to use real-time data for its machine learning models, eventually moving workflows and data streams onto cloud networks and creating closed-loop automation processes.
"Machine learning and artificial intelligence have seen significant growth in recent years," Muller said. "We are now trialing software from external companies that can optimize drilling parameters and connect directly to the rig's set points. This will increase ROP, reduce drilling time and leave a better well for completions. By partnering with external companies, we are hopeful that we can reduce the time it takes to develop the software, which will yield results faster and more efficiently."
Long-term value creation
The strategic intent behind the optimization initiative is to use holistic data sets to improve project-wide and well-specific decisions.
“The future is we want to have little building blocks along the way and then in the end, all those building blocks talk to each other,” Muller said. “It’s going to take time to create those building blocks. But that’s what we are doing right now. So far, we have a couple of drilling ones and we’re working on a couple of completion and production ones. And then at the end of the game, we want to have them all sitting on the cloud and talking to each other. That’s really what we want to do long term with this project.”

Aiming for a closed-loop process
"This is a close collaboration, not just between GCR Wells and GCR Development, but also with Global Wells and IT," said GCR Wells Engineering Manager David Limbert. "And without any one of these parties, our advancements wouldn't be possible. Cross-disciplinary and cross-functional collaboration have been crucial to the project's success."
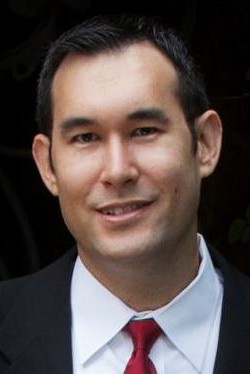
The project is closely linked with multiple initiatives within GCR, Limbert said.
"Ultimately, we are trying to lead up to a closed-loop drilling and completion process," Limbert said. "And we are also trying to link the well construction aspects back in with how we operate and produce these wells to ensure that we are delivering the best value for the company's investment."
ConocoPhillips is exploring how to leverage the project's modeling capabilities and workflows in other parts of its business, such a predicting failures in artificial lift equipment, optimizing completion designs and predicting reservoir performance (by identifying depleted reservoirs).